Translate this page into:
Risk Factors for Mortality Among Patients on Hemodialysis in India: A Case-Control Study
Corresponding author: Suresh Sankarasubbaiyan, NephroPlus Dialysis Centers, Hyderabad, Telangana, India. Email: suresh150902@gmail.com
-
Received: ,
Accepted: ,
How to cite this article: Sankarasubbaiyan S, Pollock CA, Anandh U, Kasiviswanathan S, Shah KD. Risk Factors for Mortality Among Patients on Hemodialysis in India: A Case-Control Study. Indian J Nephrol. doi: 10.25259/ijn_563_23
Abstract
Background
Mortality in hemodialysis (HD) exceeds that of many solid organ cancers, despite advancements in care. This study was conducted to understand mortality in a large dialysis network of Indian HD patients and attempt to elucidate risk factors for mortality.
Materials and Methods
We performed a case-control study of deaths among patients undergoing HD across 203 centers in India from January 1 to March 31, 2021 with an age-matched control of survivors. We reviewed demographic, dialysis, clinical, and socioeconomic factors.
Results
Out of 17,659 patients on dialysis, 554 cases (non-survivors) and 623 age-matched controls (survivors) patients were followed up. The mean age was 54.9 ± 13.8 years, 70.5% (391) of them were males. Gender, BMI, dialysis frequency, HD vintage time, history of diabetes, and heart failure were similar between non-survivors and survivors. Lower education level, payment under public insurance, dialysis facility under a public-private partnership, vascular access via catheter, hemoglobin <8 g/dL levels, serum albumin <3.5 g/dL were significantly higher, hospitalizations in 3 months prior to death were more frequent among non-survivors.
Conclusion
Factors including Hb <8 g/dL, temporary catheter, serum albumin less <3.5 g/dL, lower educational status, and dialysis under public insurance are associated with poorer survival in our population. Our findings have implications for designing interventions needed to improve outcomes for evolving policy and public-payer systems.
Keywords
Hemodialysis
End-stage renal disease
Chronic kidney disease
Mortality
Risk factors
Introduction
Hemodialysis (HD) is the dominant form of renal replacement therapy (RRT) worldwide, with survival of 93% at 1 year and 79% at 5 years in the 45–64 years age group in the ANZDATA registry.1 The USRDS 2020 annual data report showed that incident patients who commenced HD in 2013 had 1-year survival of 97.5% and 5-year survival of 88.5%.2 Among middle-income countries, the Malaysian renal registry reported a 1-year survival of 88%, 3-year survival of 68%, and 5-year survival of 53%.3 Dialysis Outcomes Practice Patterns Study (DOPPS) data in developed countries demonstrate that mortality is three times higher in the initial 3 months compared to a later period.4 In India, approximately 9% to 13% of patients on HD die within 1 year.5 Predictors of mortality in HD patients include dialysis duration, comorbidity, socioeconomic, and education status even in developed countries.6,7 In the Indian context, HD has grown with improving public insurance payments, resulting in improved access for patients with poor socioeconomic status as illustrated in a recent review that highlighted the challenges in dialysis delivery in India.6 Few single-center studies in India have reported survival on HD and have rarely looked into the risk factors responsible for mortality.8 Our study was undertaken to understand mortality in a large dialysis network of Indian HD patients and to elucidate risk factors for mortality.
Materials and Methods
We performed a case-control study among prevalent patients undergoing maintenance HD (MHD) in a single large dialysis network across India. In 203 centers for HD, spread across 22 states, 17,659 (sampling frame) patients were registered from January 1 to March 31, 2021.
Selection of cases
All patients on MHD who expired from non-covid etiology from January to March 2021.
Criteria for selecting controls
Patients on maintenance hemodialysis (MHD) who survived during the same period from the same sampling frame by simple random sampling method. A total of 797 cases (non-survivors) and 797 controls (survivors) were selected in 1:1 basis. To avoid confounding factors, we considered age group or age frequency factor and the control subjects were categorized into age groups: 18–40 years, 41–60 years, and those over 60 years old. We excluded patients who were on HD for less than 90 days to avoid transition factors. We also excluded patients who were less than 18 years of age and arrived at the final number of 554 cases and 623 controls which still satisfies the 1:1 ratio (1:1.12) [Figure 1]. There were only nine COVID deaths during the study period. All deaths amongst cases during the study period were compared against age-stratified matched surviving HD patients (controls) for the following factors: demographic, dialysis, clinical, and socioeconomic factors. The demographic parameters included age, gender, educational qualification, and type of payer for dialysis: categorized as public insurance, out-of-pocket payment, and private insurance. The facility type was categorized as a public-private partnership or as private ownership. Dialysis factors included HD vintage, frequency of HD sessions per week as per the number of sessions done, type of vascular access and dialysis adequacy, hemoglobin level, use of erythropoietin stimulating agent, and serum albumin. We could not measure the urine output of patients on dialysis. The cause of chronic kidney disease (categorized as diabetic and non-diabetic), co-morbidities: hypertension, history of heart failure, and hospitalization in the previous 3 months were recorded. Data were collected retrospectively from hospital management information systems and electronic medical records. The ethics committee approval was waived for this study by the Institutional Ethics Committee of Nephrocare since we have used deidentified data of patients who voluntarily consented to use their information for clinical research at the time of registration at NephroPlus, even before data was collected.
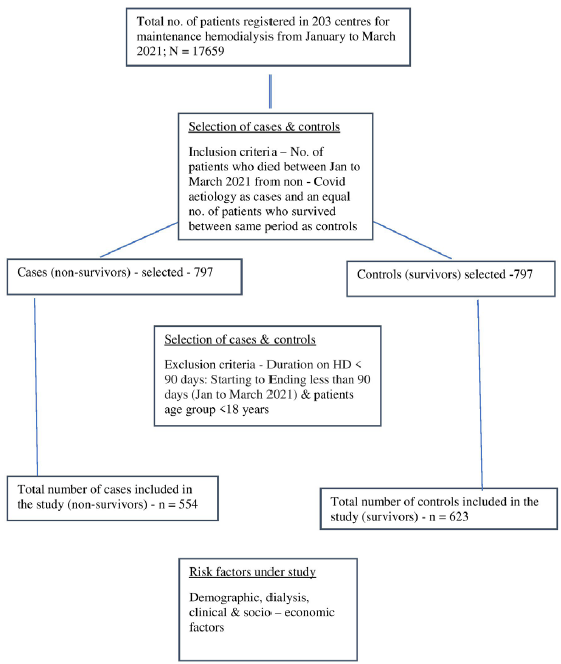
- Methodology for selection of cases and controls for the study. HD: Hemodialysis
Statistical analysis
All cases and controls were selected by stratification into 18–40 years, 41–60 years, and more than 60 years [Table 1]. All continuous variables are reported as mean and ± standard deviation (SD), remaining variables in number (n) and proportions (%). The differences between groups were tested using an independent sample t-test. An initial Chi-square test was applied to compare all variables (risk factors) between survivors and non-survivors. The univariate and multivariate logistic regression was performed to calculate the risk of mortality in odds ratio with a 95% confidence interval. All variables were tested for univariate analysis and significant variables with p-value < 0.05 were tested for multivariate analysis. To analyze the data SPSS (IBM SPSS Statistics for Windows, Version 26.0, Armonk, NY: IBM Corp. Released 2019) was used. The significance level was fixed at 5% (α = 0.05).
Group count | Total | ||
---|---|---|---|
Cases | Controls | ||
Age in years | |||
18 to 40 years | 76 | 89 | 165 |
41 to 60 years | 274 | 307 | 581 |
>60 years | 204 | 227 | 431 |
Total | 554 | 623 | 1177 |
Results
Among patients dialyzed across 203 centers, 554 patients who died from non-COVID etiologies were included as cases and 623 survivors as controls during the study period.
The comparison of demographic, dialysis, clinical, and socioeconomic characteristics between non-survivors and survivors is shown in Table 2. Non-survivors were more likely to be illiterate (56.1% vs. 43.9%, p < 0.01). Age, gender, and BMI were not significantly different between the groups. Non-survivors had a higher proportion of central venous catheters during the 3 months study period (55.8% vs. 44.2%, p < 0.05), and lower hemoglobin (mean 8.77 SD ± 1.5, p < 0.001) and lower serum albumin (albumin <3.5 g%, mean 3.44 SD ± 0.6, p < 0.001) than survivors. The distribution of HD frequency and vintage time on HD was similar between the groups. Non-survivors were more likely to be paid by public insurance (59.6% vs. 40.4%, p < 0.001) and dialyzed in centers under public-private partnership (56.7% vs. 43.3%, p < 0.001), more likely to have diabetes (51.1% vs. 48.9%, p < 0.05) with prior history of heart failure (58.4% vs. 41.6%, p < 0.05) and prior hospitalization (74% vs. 26%, p < 0.001).
Characteristics |
Non-survivors (n = 554) n (%) |
Survivors (n = 623) n (%) |
p-value |
---|---|---|---|
Demographic factors | |||
Age group (years) | 55.57 ± 13.4 | 54.77 ± 13.1 | 0.303 |
Gender | 0.688 | ||
Male | 391 (47.5) | 433 (52.5) | |
Female | 163 (46.2) | 190 (53.8) | |
Education | <0.001 | ||
Illiterate | 197 (56.1) | 154 (43.9) | |
High school | 171 (49.9) | 172 (50.1) | |
Higher Secondary | 89 (43.4) | 116 (56.6) | |
College | 97 (34.9) | 181 (65.1) | |
BMI (Indian) | 22.35 ± 4.7 | 22.88 ± 4.6 | 0.050 |
Dialysis factors | |||
HD duration (months) | 21.76 ± 16.1 | 24.72 ± 18.1 | 0.003 |
HD frequency per week | |||
1X | 14 (60.9) | 9 (39.1) | 0.281 |
2X | 282 (45.5) | 338 (54.5) | |
3X | 254 (48.7) | 268 (51.3) | |
Irregular/SOS | 4 (33.3) | 8 (66.7) | |
Vascular access | 0.011 | ||
AVF/AVG | 453 (45.5) | 543 (54.5) | |
Catheter | 101 (55.8) | 80 (44.2) | |
Dialysis adequacy | 1.25 ± 0.1 | 1.26 ± 0.1 | 0.336 |
Clinical factors | |||
Hemoglobin level g/dL | 8.77 ± 1.5 | 9.33 ± 1.5 | 0.000 |
Serum albumin g/dL | 3.44 ± 0.6 | 3.62 ± 0.4 | 0.000 |
EPO use | 0.139 | ||
Yes | 536 (47.5) | 592 (52.5) | |
No | 18 (36.7) | 31 (63.3) | |
Cause of CKD | 0.042 | ||
Non-diabetic | 343 (44.9) | 421 (55.1) | |
Diabetic | 211 (51.1) | 202 (48.9) | |
Hypertension | |||
Systolic BP in mmHg | 153.42 ± 26.3 | 149.98 ± 20.4 | 0.012 |
Diastolic BP in mmHg | 83.13 ± 12.2 | 82.78 ± 11.1 | 0.614 |
History of heart failure | <0.039 | ||
Yes | 45 (58.4) | 32 (41.6) | |
No | 509 (46.3) | 591(53.7) | |
Hospitalization in previous 3 months | <0.001 | ||
Yes | 202 (74) | 71 (26) | |
No | 352 (38.9) | 552 (61.1) | |
Socioeconomic factors | |||
Payer type | <0.001 | ||
Out of pocket | 150 (43.5) | 195 (56.5) | |
Private insurance | 118 (32.7) | 243 (67.3) | |
Public insurance | 273 (59.6) | 185 (40.4) | |
Mixed | 13 (100) | 0 | |
Type of center | <0.001 | ||
Public-private | 283 (56.7) | 216 (43.3) | |
Private hospital-based | 271 (40) | 407 (60) |
*Continuous variables reported as mean ± standard deviation. P>0.05 is considered as statistically significant. The values highlighted in bold are statistically significant. BMI: Body Mass Index; HD: Hemodialysis; SOS: When needed; AVF: Arteriovenous Fistula; AVG: Arteriovenous Graft; EPO: Erythropoietin; BP: Blood pressure; CKD: Chronic Kidney Disease
On multiple logistic regression shown in Table 3, illiteracy had an AOR of 2.68 (1. 61, 4.48) p < 0.001 and high school education AOR 1.85 (1.21, 2.85), p < 0.05 for risk of mortality compared to the college group. Patients under public insurance had an AOR: 1.93 (1.08, 3.46), p < 0.05 and patients dialyzed under a public-private partnership had an AOR 2.32 (1.38,3.92), p < 0.05 for mortality compared to those with out-of-pocket payment. Among dialysis factors, the presence of temporary vascular access had AOR 1.53 (0.99, 2.39), p < 0.05, hemoglobin less than 8 g% had AOR 1.85 (1.13, 3.04) p < 0.05, and serum albumin less than 3.5 g/dL had AOR 1.98 (1.42, 2.77), p < 0.05. There was no difference in dialysis duration between groups. Hospitalization within the previous 3 months had AOR 0.18 (0.12, 0.27), p < 0.001. Though the comparison of proportions between cases and controls for factors such as the cause of CKD – diabetes and history of heart failure were significant, they were not significant on multiple logistic regression as mortality predictors.
Characteristics | OR (95% CI) | p-value | AOR (95% CI) | p-value |
---|---|---|---|---|
Demographic factors | ||||
Age (years) | ||||
18–40 | Ref | |||
41–60 | 1.045 (0.739, 1.478) | 0.803 | ||
>60 | 1.052 (0.734, 1.508) | 0.781 | ||
Gender | ||||
Female | Ref | |||
Male | 1.053 (0.820, 1.351) | 0.688 | ||
Education status | ||||
College | Ref | |||
Illiterate | 2.387 (1.726, 3.302) | <0.001 | 2.689 (1.613, 4.483) | 0.000 |
High school | 1.855 (1.340, 2.568) | <0.001 | 1.859 (1.212, 2.851) | 0.005 |
Higher Secondary | 1.432 (.989, 2.073) | 0.058 | 1.450 (0.909, 2.313) | 0.118 |
BMI | ||||
Obese (≥25.0) | Ref | |||
Underweight (<18.5) | 1.264 (0.883, 1.809) | 0.201 | ||
Normal (18.5–22.9) | 1.109 (0.825, 1.490) | 0.494 | ||
Overweight (23– 24.9) | 1.081 (0.752, 1.552) | 0.674 | ||
Dialysis factors | ||||
HD duration (months) | ||||
>36 | Ref | |||
4–12 | 1.421 (1.037, 1.948) | 0.029 | 1.338 (0.850, 2.105) | 0.208 |
13–24 | 1.131 (0.817, 1.566) | 0.458 | 1.224 (0.769, 1.948) | 0.395 |
25–36 | 1.057 (0.719, 1.553) | 0.779 | 1.221 (0.708, 2.106) | 0.473 |
HD frequency per week | ||||
2X | Ref | |||
1X | 1.864 (0.795, 4.372) | 0.152 | ||
3X | 1.136 (0.900, 1.435) | 0.284 | ||
Irregular/SOS | 0.599 (0.179, 2.011) | 0.407 | ||
Vascular access | ||||
AVF/AVG | Ref | |||
Catheter | 1.513 (1.100, 2.081) | 0.011 | 1.539 (0.990, 2.392) | 0.045 |
Dialysis adequacy | ||||
≥1.2 | Ref | |||
<1.2 | 1.513 (0.969, 2.361) | 0.068 | ||
Clinical factors | ||||
Hb level (g/dL) | ||||
10–12 | Ref | |||
>12 | 1.003 (0.474, 2.124) | 0.993 | 1.187 (0.808, 1.745) | 0.383 |
8–9.9 | 1.470 (1.113, 1.941) | 0.007 | 1.224 (0.451, 3.323) | 0.691 |
<8 | 2.697 (1.915, 3.798) | <0.001 | 1.859 (1.137, 3.041) | 0.014 |
Serum albumin (g/dL) | ||||
≥3.5 | Ref | |||
<3.5 | 2.569 (1.940, 3.401) | <0.001 | 1.986 (1.424, 2.770) | 0.000 |
EPO use | ||||
Yes | Ref | |||
No | 0.641 (0.355, 1.160) | 0.142 | ||
Cause of CKD | ||||
Non-diabetic | Ref | |||
Diabetic | 1.282 (1.009, 1.630) | 0.042 | 1.362 (0.972, 1.911) | 0.073 |
HTN | ||||
No | Ref | |||
Yes | 0.922 (0.698, 1.218) | 0.568 | ||
History of heart failure | ||||
No | Ref | |||
Yes | 0.612 (0.383, 0.978) | 0.040 | 0.620 (0.325, 1.185) | 0.148 |
Hospitalization in previous 3 months | ||||
No | Ref | |||
Yes | 0.224 (0.166, 0.303) | <0.001 | 0.189 (0.129, 0.277) | 0.000 |
Socioeconomic factors | ||||
Payer type | ||||
Out of pocket | Ref | |||
Private insurance | 0.631 (0.465, 0.857) | 0.003 | 0.643 (0.440, 0.941) | 0.063 |
Public insurance | 1.918 (1.445, 2.546) | <0.001 | 1.936 (1.083, 3.461) | 0.026 |
Mixed | 21.706 | 0.998 | 16.65 | 0.998 |
Type of center | ||||
Private hospital | Ref | |||
Public-private | 1.968 (1.556, 2.488) | <0.001 | 2.327 (1.381, 3.920) | 0.002 |
OR: Odds Ratio; CI: Confidence Interval; AOR: Adjusted Odds Ratio; BMI: Body Mass Index; HD: Hemodialysis; SOS: When needed; AVF: Arteriovenous Fistula; AVG: Arteriovenous Graft; Hb: Hemoglobin; EPO: Erythropoietin; CKD - Chronic Kidney Disease; HTN: Hypertension. P value < 0.05 is considered as statistically significant.
Discussion
This study documents the important factor associated with the mortality on HD in India. Globally, race, temporary vascular access, dialysis adequacy, interdialytic interval, dialysis vintage time, nutritional status, diabetes, facility-doctor-patient ratio, and socioeconomic factors are known to influence survival on dialysis.9,10
The increased mortality risk of central venous catheter use is consistent with previous large observational studies of the dialysis population. Among 5507 prevalent dialysis patients, arteriovenous graft (AVG) had a relative risk (RR) of 1.41 (p < 0.05) and catheter had an RR of 1.54 (p < 0.05) for all-cause mortality, infection-related, and cardiac mortality among patients with and without diabetes similarly.11 Among incident dialysis patients in the CHOICE study, the adjusted hazard ratio for mortality was 1.5 among patients using dialysis catheters compared to arteriovenous fistula (AVF).12 We did not study specific catheter complications, including infection, catheter malfunction, and hospitalizations.
The impact of anemia on survival in HD patients was demonstrated in a DOPPS study where case-mix adjusted mortality risk and hospitalization decreased incrementally by 5% and 6% respectively, for every gram of increase in hemoglobin.13 In our study, 47% of non-survivors had hemoglobin between 8 and 9.9 g/dL, and 61.7% had hemoglobin less than 8 g/dL with an AOR: 1.85 (1.13, 3.04 2.95). It likely reflects a suboptimal dose of erythropoietin and iron due to “out-of-pocket” payment and the lack of bundled payments under public insurance. Previous studies among the Indian HD population showed a high prevalence of anemia.8,14,15 Significantly, in India, the general population has a high prevalence of anemia with 57% and 25% of women and men, respectively, in the age group of 15 to 49 years as reported in the recent National Family Health Survey.16
In an analysis of the HEMO study, 12 nutritional indicators, including low body mass index and lower serum albumin were associated with lower survival in less than 6 months adjusted for case mix, comorbidity, and type of dialyzer.17 Similarly, in the DOPPS study, variations of nutritional indicators, including serum creatinine, serum albumin, BMI, and normalized protein catabolic rate were independently associated with poorer survival. These factors were also demonstrated to have a multiplicative effect on survival.18 In our study, non-survivors were more likely to have serum albumin less than 3.5 g/dL and were associated with higher AOR 1.98 (1.42, 2.77) for death.
In our study population, 51%–54% were on twice weekly HD and 3% once weekly HD. Twice weekly HD preserves residual renal function and potentially improves AVF longevity and better quality of life against the risks of lesser solute clearance, weight control, and electrolyte imbalance.19 Twice weekly HD could potentially be used for the initial phase of HD in low-resource settings, it is practiced more by patient choice, economic compulsion, and payer preferences too.8,14,15 We did not document the patients’ residual renal function for twice weekly HD. The small sample size and other confounders could be a limiting factor in demonstrating the lack of influence of HD frequency on survival.
The impact of socioeconomic factors on survival on hemodialysis across racial groups has been illustrated by an analysis of USRDS data.20,21 In the USA, segregated neighborhoods among blacks and lower median income among whites showed increased mortality risk.20 In our population, patients under public insurance form a large proportion, and this is reported to be the most rapidly growing segment of the dialysis population in India.22 An analysis of insurance claims data from one state in India shows a drop-out rate of 36% in the first 6 months and a median survival of 170 for females to 198 days for males.23 Despite government subsidies, the out-of-pocket burden on families for expenditure on dialysis is considerable at 27,564 Indian rupees, constituting up to 80% of household expenditure.24 The incremental steps are required to lessen the cost burden resulting from biochemical monitoring access interventions and ancillary medications.
Hospitalization within 3 months had a highly significant AOR on mortality. It is well known that hospitalizations lead to high healthcare costs and result from infection, fluid overload, and cardiac and psychosocial comorbidity.25 A DOPPS study of five European countries showed that hospitalization was significantly associated with the recent start of HD particularly in the settings of diabetes, cardiac disease, peripheral vascular disease, recent gastrointestinal (GI) bleeding, and psychiatric disease.26 The presence of an irreversible chronic illness coupled with economic hardships is often responsible for skipping medications and dialysis. Missing dialysis often increases hospitalization and mortality.27 Missed treatments had a positive association with all-cause and cardiovascular mortality and hospitalization.28 In our context, dialysis factors including anemia, temporary vascular access, and low serum albumin level, combined with socioeconomic factors reflected in educational status, dependence on public insurance and therefore hurdles to access acute care could be drivers of hospitalization.
Our study’s strength lies in its unique insights from a large dialysis network, highlighting health system challenges—both medical and non-medical—during the development of end-stage renal disease programs in lower-middle-income countries. Interventions focused on improving anemia, access management, and nutrition combined with education and support for the socio-economically challenged and poorly educated are essential to improve outcomes.
The limitations of this study are its retrospective nature, absence of data regarding blood pressure control, fluid management, other comorbidities particularly infections, objective cardiac functional assessment, residual renal function, access complications, and reasons for hospitalizations. The lab data were assessed from physical records at any time during the 3 months of the study period owing to the widely spread network of dialysis centers, lack of digital data, and limited ability to collect all data retrospectively.
In India, since the government is funding treatment for kidney failure, this study provides a roadmap to improve outcomes for patients commencing RRT beyond the provision of dialysis.
Conclusion
Factors such as lower educational status, vascular access using temporary catheters, hemoglobin level less than 8 g/dL, serum albumin less than 3.5 g/dL, hospitalization in previous 3 months, and dialysis using public insurance are associated with poor survival in our population. Hence, pre-dialysis care, planning for dialysis treatments, and early detection interventions are important to improve dialysis outcomes and to limit high-cost RRT in all jurisdictions.
Acknowledgments
The data were collected from various NephroPlus Dialysis Centres across India.
Conflicts of interest
There are no conflicts of interest.
References
- Available from: https://www.anzdata.org.au/report/anzdata-44th-annual-report-2021-data-to-2020/ [Accessed 18 Sep 2023].
- Epidemiology of kidney disease in the United States. Am J Kidney Dis. 2021;77:A7-A8.
- [CrossRef] [PubMed] [Google Scholar]
- Malaysian society of nephrology. Kuala Lumpur; Available from: https://www.msn.org.my/nrr/mdtr2016.jsp [Accessed 18 Sep 2023].
- Worldwide, mortality risk is high soon after initiation of hemodialysis. Kidney Int. 2014;85:158-65.
- [CrossRef] [PubMed] [PubMed Central] [Google Scholar]
- Survival and analysis of predictors of mortality in patients undergoing hemodialysis in a rural hospital in Puducherry, India: A retrospective cohort study. Open Urol Nephrol J. 2023;16
- [Google Scholar]
- Extended-hours hemodialysis is associated with lower mortality risk in patients with end-stage renal disease. Kidney Int. 2016;90:1312-20.
- [CrossRef] [PubMed] [PubMed Central] [Google Scholar]
- Poverty and mortality in hemodialysis patients. J Am Soc Nephrol. 2013;24:165-7.
- [CrossRef] [PubMed] [Google Scholar]
- Challenges and limitations of maintenance hemodialysis in urban South India. Hemodial Int. 2007;11:485-91.
- [CrossRef] [PubMed] [Google Scholar]
- Early outcomes among those initiating chronic dialysis in the United States. Clin J Am Soc Nephrol. 2011;6:2642-9.
- [CrossRef] [PubMed] [PubMed Central] [Google Scholar]
- Predictors of early mortality among incident US hemodialysis patients in the dialysis outcomes and practice patterns study (DOPPS) Clin J Am Soc Nephrol. 2007;2:89-99.
- [CrossRef] [PubMed] [Google Scholar]
- Type of vascular access and mortality in U.S. hemodialysis patients. Kidney Int. 2001;60:1443-51.
- [CrossRef] [PubMed] [Google Scholar]
- Identifying individuals with a reduced GFR using ambulatory laboratory database surveillance. J Am Soc Nephrol. 2005;16:1433-9.
- [CrossRef] [PubMed] [Google Scholar]
- Anemia management and outcomes from 12 countries in the dialysis outcomes and practice patterns study (DOPPS) Am J Kidney Dis. 2004;44:94-111.
- [CrossRef] [PubMed] [Google Scholar]
- End-stage renal disease patients on hemodialysis: A study from a tertiary care center in a developing country: Hemodialysis in a developing country. Hemodial Int. 2011;15:312-19.
- [CrossRef] [PubMed] [Google Scholar]
- Survival analysis of patients on maintenance hemodialysis. Indian J Nephrol. 2014;24:206-13.
- [CrossRef] [PubMed] [PubMed Central] [Google Scholar]
- Compendium of fact sheets. Ministry of Health and Family Welfare. Government of India. Available from: https://main.mohfw.gov.in/sites/default/files/NFHS-5_Phase-II_0.pdf [Accessed 18 Sep 2023].
- Are nutritional status indicators associated with mortality in the hemodialysis (HEMO) Study? Kidney Int. 2005;68:1766-76.
- [CrossRef] [PubMed] [Google Scholar]
- Independent and joint associations of nutritional status indicators with mortality risk among chronic hemodialysis patients in the dialysis outcomes and practice patterns study (DOPPS) J Ren Nutr. 2010;20:224-34.
- [CrossRef] [PubMed] [Google Scholar]
- Twice-weekly hemodialysis in China: Can it be A better option for initiation or maintenance dialysis therapy? Semin Dial. 2017;30:277-81.
- [CrossRef] [PubMed] [PubMed Central] [Google Scholar]
- Segregation, income disparities, and survival in hemodialysis patients. J Am Soc Nephrol. 2013;24:293-301.
- [CrossRef] [PubMed] [PubMed Central] [Google Scholar]
- Neighborhood socioeconomic status, race, and mortality in young adult dialysis patients. J Am Soc Nephrol. 2014;25:2649-57.
- [CrossRef] [PubMed] [PubMed Central] [Google Scholar]
- Available from: https://nhsrcindia.org/ [Accessed 08 Jul 2024], P.M.N.D. National Health Systems Resource Centre. Nhsrcindia.org. [Accessed 17 July 2023].
- Utilization, costs, and outcomes for patients receiving publicly funded hemodialysis in India. Kidney Int. 2018;94:440-5.
- [CrossRef] [PubMed] [Google Scholar]
- Paying for hemodialysis in Kerala, India: A description of household financial hardship in the context of medical subsidy. Kidney Int Rep. 2019;4:390-8.
- [CrossRef] [PubMed] [PubMed Central] [Google Scholar]
- Decreasing hospitalizations in patients on hemodialysis: Time for a paradigm shift. Semin Dial. 2018;31:278-88.
- [CrossRef] [PubMed] [Google Scholar]
- Mortality and hospitalization in hemodialysis patients in five European countries: Results from the dialysis outcomes and practice patterns study (DOPPS) Nephrol Dial Transplant. 2004;19:108-20.
- [CrossRef] [PubMed] [Google Scholar]
- Identification of volume overload hospitalizations among hemodialysis patients using administrative claims: A validation study. BMC Nephrol. 2016;17:173.
- [CrossRef] [PubMed] [PubMed Central] [Google Scholar]
- Missed hemodialysis treatments: International variation, predictors, and outcomes in the dialysis outcomes and practice patterns study (DOPPS) Am J Kidney Dis. 2018;72:634-43.
- [CrossRef] [PubMed] [Google Scholar]