Translate this page into:
Comparative Analysis of Tools for Assessment of Protein–Energy Wasting in Chronic Kidney Disease Patients on Maintenance Hemodialysis
Corresponding author: Dr. Ramasubramanian Viswanathan, Department of Nephrology, Tirunelveli Medical College and Hospital, Tirunelveli, Tamil Nadu, India. E-mail: nephpubtvmch@gmail.com
-
Received: ,
Accepted: ,
How to cite this article: Sivagnanam H, Senthilkumar PK, Velu KB, Anand M, Viswanathan R. Comparative Analysis of Tools for Assessment of Protein–Energy Wasting in Chronic Kidney Disease Patients on Maintenance Hemodialysis. Indian J Nephrol. 2024;34:453-60. doi: 10.4103/ijn.ijn_57_23
Abstract
Background:
Patients with chronic kidney disease have muscle wasting, sarcopenia, and cachexia that contribute to frailty and morbidity. The present study assessed the prevalence of protein–energy wasting in dialysis-dependent chronic kidney disease population and evaluated the validity of various nutritional assessment tools in diagnosing protein–energy wasting.
Materials and Methods:
All patients above 18 years undergoing dialysis for more than 3 months without any active infection or malignancy were included in our study. Data from anthropometric measurements, dietary assessment, and blood investigations were collected. Protein–energy wasting was assessed by the International Society of Renal Nutrition and Metabolism 2008 criteria. Diagnostic validity of the nutritional assessment tools to predict protein–energy wasting was estimated by area under the curve, sensitivity, specificity, and accuracy statistics.
Results:
A total of 146 patients were studied. The prevalence of protein–energy wasting was 56.8%. Protein–energy wasting was significantly associated with socioeconomic status, hospitalization days, and catheter days. Normalized protein catabolism rate had the highest sensitivity (90.4%) for predicting protein–energy wasting. Malnutritional inflammatory score had the highest area under the curve (0.858), specificity (82.5%), and accuracy (82.2%). Mid-upper arm circumference, Dialysis Malnutrition Score, and albumin were also found to be significant predictors of protein–energy wasting.
Conclusion:
Lack of advanced equipment in suburban and rural centers to detect protein–energy wasting in India can be overcome by using the various stand-alone and combination nutrition assessment tools which have been validated in the present study.
Keywords
Chronic kidney disease
Maintenance hemodialysis
Malnutrition
Malnutrition Inflammation Score
Nutritional assessment
Protein–energy wasting
Introduction
Protein–energy wasting (PEW) is a state of disordered catabolism resulting from metabolic and nutritional derangements in chronic disease states. Patients with chronic kidney disease (CKD), and end-stage renal disease (ESRD) in particular, have muscle wasting, sarcopenia, and cachexia, contributing to frailty and morbidity. A meta-analysis conducted by the International Society of Renal Nutrition and Metabolism (ISRNM) in 2018, which included an Indian study, estimated that the prevalence of PEW varies among countries and dialysis centers (around 18%–64%).1 It also observed that the evaluation of PEW is hampered by a lack of standardized PEW definitions, variability of existing assessment tools, and differences in socioeconomic status (SES) among the countries. Thus, establishing proper screening tools was considered an important starting point.1 Another meta-analysis conducted in 2021 estimated the worldwide prevalence of PEW to be from 35.2% to 50.6% and the prevalence in India as 56.7% based on nine studies.2 Eight studies used Subjective Global Assessment (SGA) to assess the prevalence and one used Malnutrition Inflammation Score (MIS).2 There is no accepted definition available for PEW. In 2008, ISRNM established readily usable criteria for PEW, which required multiple parameters for diagnosis.3 Henceforth, various nutritional assessment tools and questionnaires have been developed aiming at rapid and effective diagnosis of PEW. However, various studies comparing the efficacy of various tools yield variable results. There is also a considerable lack of Indian data in this regard. Most Indian dialysis centers lack advanced equipment like body composition monitor and bioimpedance for nutritional assessment. So, effective alternate nutritional tools are necessary to screen/diagnose and consequently treat PEW in the Indian CKD population. The present study was undertaken to assess the prevalence of PEW in the dialysis-dependent CKD population and to determine the diagnostic validity of various nutritional assessment tools in diagnosing PEW.
Materials and Methods
The present study was a cross-sectional, observational, validation study conducted over 3 months in a tertiary care hospital in southern Tamil Nadu from June to September 2022. The eligibility criteria and the methods applied were based on the 2020 Kidney Disease Outcome Quality Initiative (KDOQI) clinical practice of nutrition guidelines.4 Patients above 18 years of age who had been on hemodialysis for at least 3 months and signed informed written consent were included in the study. Patients with acute infection at the time of presentation, patients with temporary access catheter, patients with malignancy at the time of presentation, and patients who refused to give informed written consent were excluded from the study. Predesigned, pretested, interviewer-administered semi-structured questionnaire for demographic and epidemiological data was used (SES was calculated by the modified Kuppuswamy scale).5 The study was undertaken following the process depicted in Figure 1. Ethical approval to conduct the study was obtained from the Institutional Ethics Committee of Tirunelveli Medical College and Hospital (ID no. 20222395). Eligible patients were enrolled after getting informed written consent from them.

- Sequence of the study process.
Anthropometric measurements: Patients’ body weight and height were measured using the spider 505 platform weight scale and stand-alone stadiometer, respectively. The body mass index (BMI) of patients was computed using the formula weight (kg)/(height × height) (m2) based on dry weight. A monthly weight assessment was used to determine whether the weight loss was significant. As an indicator of fluid compliance, interdialytic weight gain (IDWG) was calculated by subtracting the postdialysis weight of the previous dialysis session from the predialysis weight.6 Mid-upper arm circumference (MUAC) of the nonfistula arm was measured using a flexible, nonstretchable measuring tape at the midpoint of the upper arm, between the acromion and the olecranon process, after completion of the dialysis session. Waist–hip ratio (WHR) was assessed by taking the waist circumference and hip circumference using a measuring tape postdialysis. All measurements were taken postdialysis on three separate occasions at monthly intervals by trained dialysis technicians.
Dietary assessment was made by the 24-h dietary recall and 7-day dietary records on three separate occasions at a monthly interval. Dietary energy intake (DEI) and dietary protein intake (DPI) were calculated using this data and compared to the required DEI and DPI as per the readily usable criteria of ISRNM.3
Biochemical parameters such as blood urea, serum creatinine levels, serum lipid profile, liver function test, albumin, hemogram, C-reactive protein, total iron-binding capacity (TIBC), transferrin, and urine creatinine after dialysis (urea and creatinine before and after dialysis) were collected on three separate occasions at a monthly interval.
The above investigations were used to calculate measures such as urea reduction ratio (URR), Kt/V using Daugirdas formula,7 normalized protein catabolism rate (NPCR) using Lightman simple formula,8 and simplified creatinine index using creatinine kinetic modeling.9
Questionnaires for assessing nutrition: Nutritional adequacy was graded by Malnutrition Universal Screening Tool (MUST),10 7-point SGA score,11 Dialysis Malnutrition Score (DMS),12 and the Malnutritional Inflammatory Score (MIS).13
Pulmonary hypertension (PHT) was diagnosed with an echocardiogram by a cardiologist based on the European Society of Cardiology (ESC)/European Respiratory Society (ESC/ERS) guidelines.14
All the data collected were used to calculate the prevalence of PEW using ISRNM 2008 criteria.3 It comprises the following major categories: biochemical parameters (low albumin, low prealbumin, low serum cholesterol), low body weight (BMI of less than 23, weight loss), low total body fat (less than 10%), reduction in the muscle mass of patients (muscle wasting, decreased MUAC, appearance of creatinine), and low protein/energy intake (low DPI, low DEI).3 If the patients had minimum of three among the four major categories (with minimum of one test being positive in each major category), then they can be diagnosed with kidney disease–related PEW.3
Prevalence of PEW, factors associated with PEW, and the diagnostic validity of MUAC, MUST, SGA, DMS, MIS, NPCR, albumin, and simplified creatinine index in predicting PEW were the outcomes assessed in the study.
Analysis was conducted using IBM Statistical Package for the Social Sciences (SPSS) v26.0. Categorical variables were expressed as frequency and proportion. Mean with standard deviation was calculated for continuous variables of interest. The normality of the continuous variables was tested by the Kolmogorov–Smirnov test and was found to be not normally distributed. Hence, the Mann–Whitney test was used to test the significance of the difference in continuous variables between PEW and non-PEW patients. The significance of the association between the PEW status and the categorical variables was tested by the Chi-square test. For the tools with continuous data (MUAC, MUST, DMS, MIS, NPCR, albumin, SCI), the area under the curve (AUC) was calculated by the receiver operator characteristic (ROC) curve. Ideal cut-offs to predict PEW were obtained by Youden index for the tools which had a significant AUC. The sensitivity, specificity, and accuracy of the tests were calculated for the tools based on these cut-offs for each tool. PEW in SGA was categorized according to the category of the patients (mild malnutrition to severe malnutrition were included under PEW category). The PEW diagnosis based on the ISRNM 2008 criteria was considered the gold standard. A P value of <0.05 was considered statistically significant.
Results
A total of 146 patients were included in the study. The baseline clinical, anthropometrical, and biochemical characteristics of the included patients are enumerated in Table 1. The age of the participants was 40.9 + 12.6 years; 104 (71%) were males and 42 (29%) were females. Among the patients, 19.9% had diabetes. The mean dialysis vintage was 15.2 months. Among the patients, 77.4% belonged to a lower middle SES and 61% had PHT. The average dietary intake based on 24-h dietary recall and 7-day dietary records was 994.3 kcal of energy/36 g of protein and 1010.2 kcal of energy/40 g of protein, respectively [Table 1].
Variable | All patients | PEW | Non-PEW | |||
---|---|---|---|---|---|---|
Mean | SD | Mean | SD | Mean | SD | |
Age | 40.9 | 12.6 | 38.9 | 13.3 | 43.4 | 11.2 |
SBP | 148.8 | 25.4 | 146.3 | 27.3 | 152.2 | 22.6 |
DBP | 87.9 | 16.1 | 87.2 | 18.3 | 88.9 | 12.7 |
Dialysis vintage | 15.2 | 22.4 | 14.1 | 22.8 | 16.7 | 22.0 |
Kt/V | 1.3 | 0.4 | 1.3 | 0.5 | 1.3 | 0.4 |
Urea reduction ratio | 62.5 | 12.7 | 61.6 | 14.3 | 63.5 | 10.2 |
Mean interdialytic weight gain | 2.4 | 0.9 | 2.5 | 0.9 | 2.4 | 0.9 |
Height | 159.3 | 9.2 | 159.5 | 9.3 | 159.1 | 9.2 |
Weight | 51.0 | 10.1 | 47.4 | 9.5 | 55.8 | 9.0 |
BMI | 20.0 | 3.5 | 18.3 | 3.1 | 22.1 | 2.7 |
Lean body mass | 41.7 | 8.6 | 39.5 | 8.2 | 44.5 | 8.3 |
Mid-upper arm circumference | 19.8 | 4.6 | 18.7 | 4.5 | 21.2 | 4.2 |
Hb | 7.0 | 1.6 | 6.8 | 1.6 | 7.3 | 1.5 |
Hospitalization last month | 6.5 | 8.3 | 8.3 | 9.3 | 4.1 | 6.2 |
Hospitalization per year | 10.8 | 15.1 | 14.4 | 17.5 | 6.1 | 9.4 |
Albumin | 3.4 | 0.6 | 3.3 | 0.6 | 3.6 | 0.6 |
Prealbumin | 30.8 | 1.2 | 28.6 | 1.3 | 31.2 | 1.1 |
Cholesterol | 115.0 | 35.3 | 102.9 | 37.4 | 130.9 | 24.9 |
TGL | 111.5 | 51.1 | 97.6 | 38.8 | 129.8 | 59.3 |
HDL | 40.1 | 11.1 | 38.8 | 10.9 | 41.8 | 11.3 |
LDL | 108.7 | 28.9 | 110.2 | 26.2 | 106.7 | 32.3 |
TG:HDL ratio | 3 | 1.6 | 3 | 1.6 | 3 | 1.6 |
Catheter days | 36.0 | 18.1 | 40.7 | 19.6 | 29.8 | 13.8 |
Required dietary intake | 1256.3 | 250.0 | 1179.9 | 222.7 | 1357.0 | 250.0 |
Actual dietary intake 24 h | 994.3 | 161.6 | 947.1 | 155.0 | 1056.5 | 149.7 |
Actual dietary intake 7 days | 1012.2 | 167.1 | 961.7 | 159.0 | 1078.8 | 154.9 |
EF | 58.7 | 11.9 | 58.7 | 12.6 | 58.7 | 10.9 |
WHR | 0.9 | 0.1 | 0.9 | 0.1 | 0.9 | 0.1 |
BMI=body mass index, DBP=diastolic blood pressure, EF=ejection fraction, Hb=hemoglobin, HDL=high-density lipoproteins, LDL=low-density lipoproteins, PEW=protein–energy wasting, SBP=systolic blood pressure, SD=standard deviation, TGL=triglycerides, WHR=waist–hip ratio
PEW based on ISRNM criteria (2008)
The prevalence of PEW was 56.8%. Although most of the patients in the study were males, the proportion of PEW was higher in females (61.9%) than males (54.8%) [Supplementary Table 1].
The association of various factors related to patients, dialysis, mortality, and morbidity with PEW is presented in Table 2. Age had an inverse relationship with PEW among the patients in the study, that is, patients with PEW were significantly younger than non-PEW patients (P = 0.048). A linear regression model was run between the calorie intake and protein intake and the age of the patients. Calorie intake was found to have a significant association with the age of the patients (β = 0.170, P = 0.040). Hence, we adjusted the calorie intake of patients in a logistic regression model to ascertain the association between PEW and age and found that the significance of the association between PEW and age was not retained (P = 0.144). Data from the current study showed DEI as the maximum in lunch and DPI as the maximum in breakfast.
PEW status | n | Mean rank | P | |
---|---|---|---|---|
Age | PEW | 83 | 67.48 | 0.048 |
No PEW | 63 | 81.44 | ||
Total | 146 | |||
Height | PEW | 83 | 74.72 | 0.688 |
No PEW | 63 | 71.89 | ||
Total | 146 | |||
Weight | PEW | 83 | 57.87 | <0.001 |
No PEW | 63 | 94.10 | ||
Total | 146 | |||
BMI | PEW | 83 | 52.66 | <0.001 |
No PEW | 63 | 100.95 | ||
Total | 146 | |||
Weight loss | PEW | 83 | 72.96 | 0.858 |
No PEW | 63 | 74.21 | ||
Total | 146 | |||
Lean body mass | PEW | 83 | 63.19 | 0.001 |
No PEW | 63 | 87.08 | ||
Total | 146 | |||
Hospitalizations in the last year | PEW | 83 | 83.28 | 0.001 |
No PEW | 63 | 60.61 | ||
Total | 146 | |||
Hospitalization in the last month | PEW | 83 | 81.98 | 0.004 |
No PEW | 63 | 62.33 | ||
Total | 146 | |||
Catheter days | PEW | 83 | 84.99 | <0.001 |
No PEW | 63 | 58.36 | ||
Total | 146 | |||
WHR | PEW | 83 | 70.43 | 0.311 |
No PEW | 63 | 77.55 | ||
Total | 146 | |||
Mid-upper arm circumference | PEW | 83 | 63.05 | 0.001 |
No PEW | 63 | 87.26 | ||
Total | 146 | |||
Mean interdialytic weight gain | PEW | 83 | 74.45 | 0.755 |
No PEW | 63 | 72.25 | ||
Total | 146 | |||
TG/HDL ratio | PEW | 83 | 73.08 | 0.890 |
No PEW | 63 | 74.06 | ||
Total | 146 | |||
SBP | PEW | 83 | 68.50 | 0.098 |
No PEW | 63 | 80.09 | ||
Total | 146 | |||
DBP | PEW | 83 | 70.96 | 0.396 |
No PEW | 63 | 76.84 | ||
Total | 146 | |||
PHT | PEW | 83 | 57.87 | <0.001 |
No PEW | 63 | 94.10 | ||
Total | 146 | |||
Kt/V | PEW | 83 | 72.31 | 0.696 |
No PEW | 63 | 75.07 | ||
Total | 146 | |||
Urea reduction ratio | PEW | 83 | 72.45 | 0.730 |
No PEW | 63 | 74.89 | ||
Total | 146 |
BMI=body mass index, DBP=diastolic blood pressure, HDL=high-density lipoproteins, PEW=protein–energy wasting, PHT=pulmonary hypertension, SBP=systolic blood pressure, TGL=triglycerides, WHR=waist–hip ratio. P values set in bold indicate statistical significance
The incidence of PHT was significantly higher in PEW patients (71.1%) than non-PEW patients (47.6%) (P = 0.004). Patients with PEW had a higher incidence of hospitalization per month (P = 0.001) as well as per year (P = 0.004) [Table 2]. All four patients who died during the study period had a diagnosis of PEW (100%). Five of the 146 patients (3.4%) had permanent catheters, while 96.6% were on fistulas. All those five permanent catheter patients had PEW. Patients with PEW also had significantly higher catheter days in the past than the ones without PEW. No association was found between hepatitis C virus (HCV) status (P = 0.791), coronavirus disease 2019 (COVID-19) status (P = 0.247), and PEW status. Dialysis vintage, adequacy parameters (Kt/V and URR), and WHR did not show any significant association. Weight, BMI, lean body mass, and MUAC were significantly lower among PEW patients [Table 2].
Diagnostic validity of the nutritional assessment tools in predicting PEW
The ROC curves revealed that MUAC, DMS, MIS, NPCR, and albumin had a significant AUC for predicting PEW (P < 0.05) [Table 3, Figures 2 and 3]. MIS was found to have the highest AUC (0.858, P < 0.001). The efficacy of these tools assessed by calculating sensitivity, specificity, and accuracy has been presented in Table 4. Individually, NPCR had the highest sensitivity (90.4%), while MIS was the most specific tool (82.5%) as well as the most accurate tool to predict PEW (82.2%) [Table 4]. SGA or DMS in combination with NPCR was found to have the highest sensitivity (98.8%). MIS + NPCR had the highest diagnostic accuracy among the combination of the tools (78.1%) [Table 4].
Tool | AUC (95% CI) | P | Cut-offs |
---|---|---|---|
MUAC | 0.666 (0.577-0.755) | 0.001 | <18.5 |
MUST | 0.481 (0.386-0.576) | 0.691 | NA |
DMS | 0.776 (0.701-0.852) | <0.001 | >4 |
MIS | 0.858 (0.795-0.920) | <0.001 | >8 |
NPCR | 0.784 (0.703-0.866) | <0.001 | <1.075 |
Albumin | 0.677 (0.588-0.766) | <0.001 | <3.55 |
Simplified creatinine index | 0.530 (0.434-0.625) | 0.538 | NA |
AUC=area under the curve, CI=confidence interval, DMS=Dialysis Malnutrition Score, MIS=Malnutrition Inflammation Score, MUAC=mid-upper arm circumference, MUST=Malnutrition Universal Screening Tool, NPCR=normalized protein catabolic rate, PEW=protein–energy wasting. P values set in bold indicate statistical significance
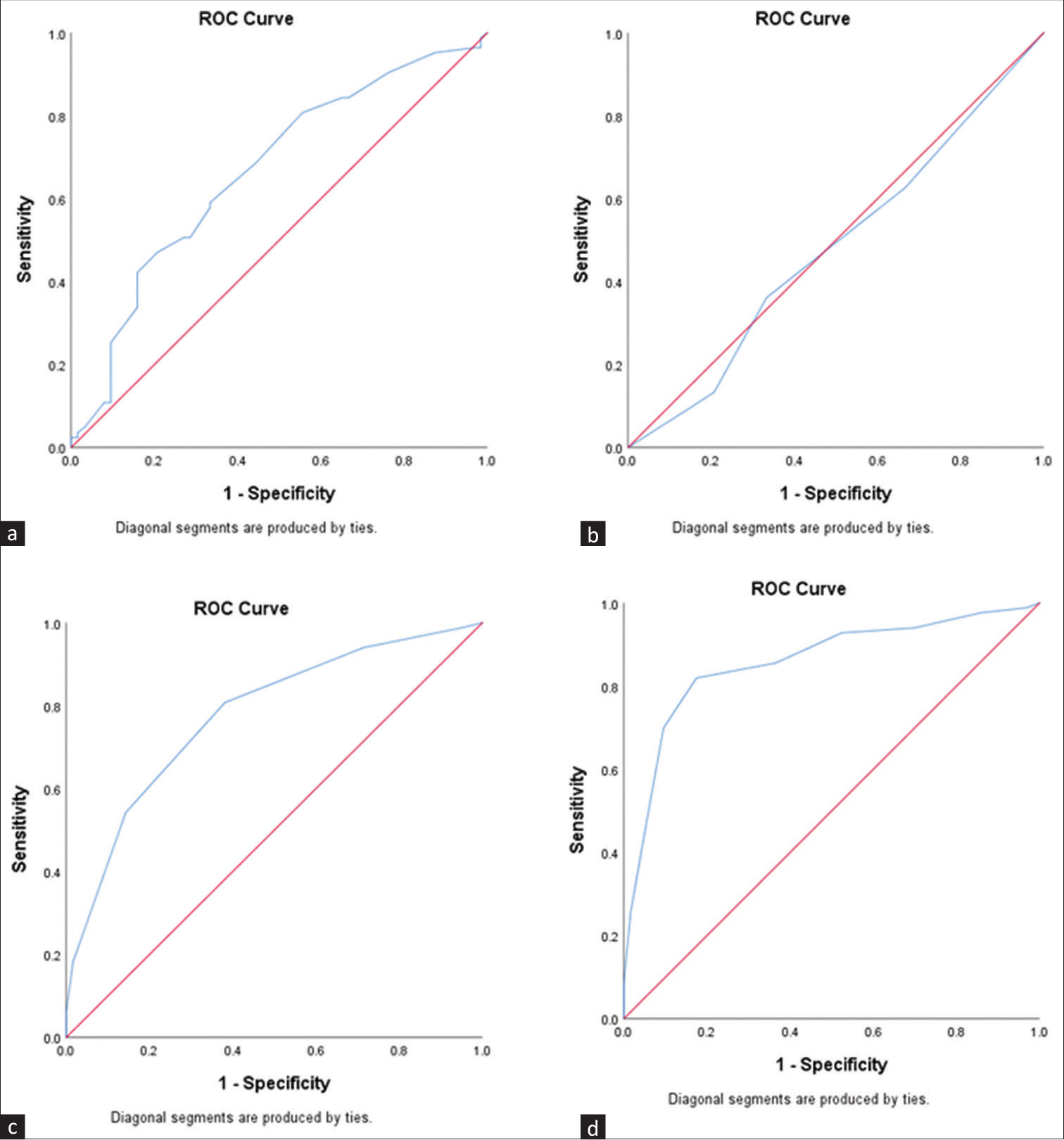
- ROC curves for predicting PEW: (a) MUAC, (b) MUST, (c) DMS, (d) MIS. DMS = Dialysis Malnutrition Score, MIS = Malnutrition Inflammation Score, MUAC = mid-upper arm circumference, MUST = Malnutrition Universal Screening Tool, PEW = protein–energy wasting, ROC = receiver operator characteristic.
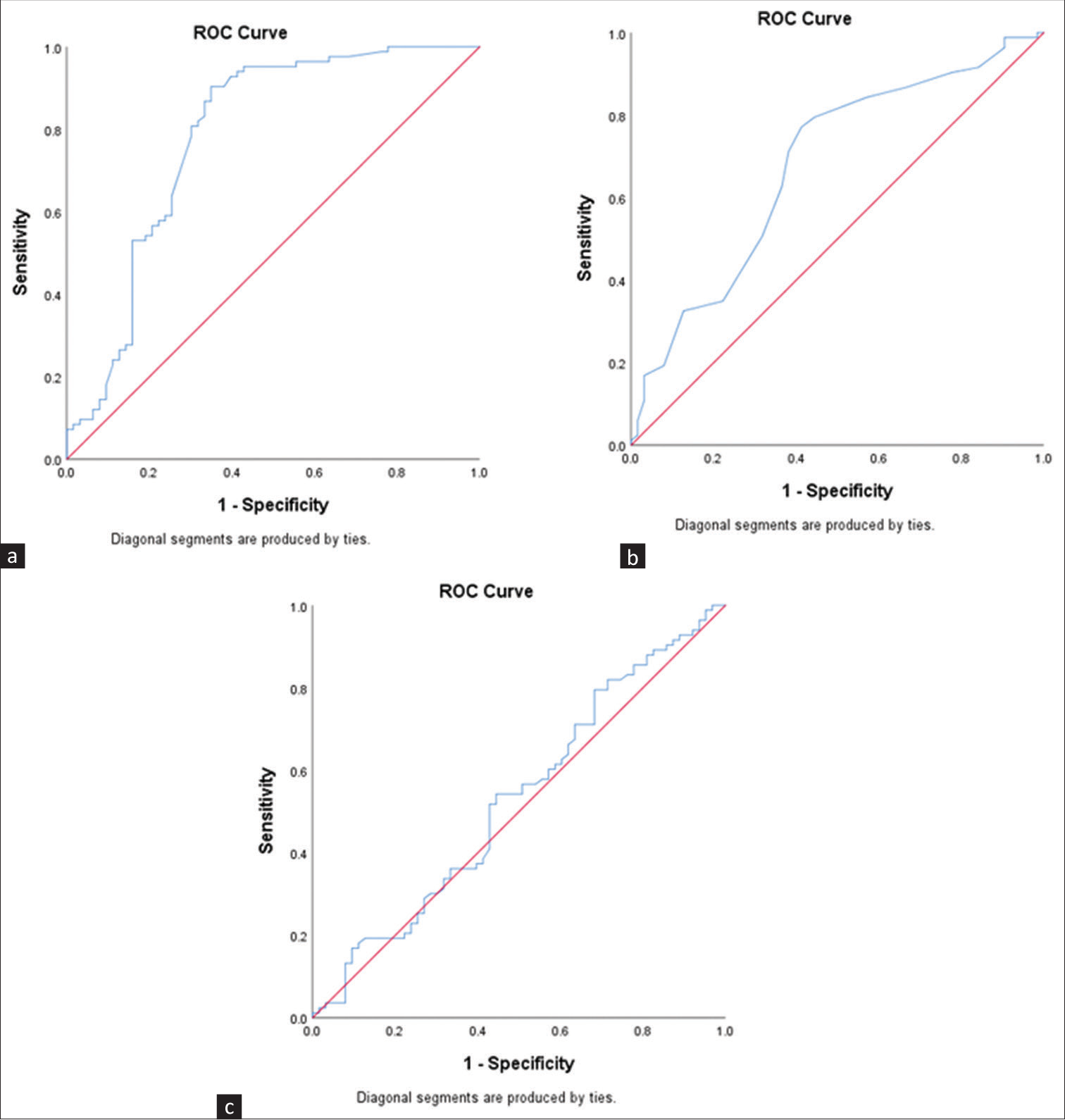
- ROC curves for predicting PEW: (a) NPCR, (b) albumin, (c) simplified creatinine index. NPCR = normalized protein catabolic rate, PEW = protein–energy wasting, ROC = receiver operator characteristic.
Tool | Sensitivity (%) | Specificity (%) | Accuracy (%) |
---|---|---|---|
MUAC | 47 | 79.4 | 62.2 |
SGA | 71.1 | 69.9 | 55.4 |
DMS | 80.7 | 61.9 | 72.6 |
MIS | 81.9 | 82.5 | 82.2 |
NPCR | 90.4 | 65.1 | 79.5 |
Albumin | 77.1 | 58.7 | 69.2 |
SGA + NPCR | 98.8 | 17.5 | 63.7 |
DMS + NPCR | 98.8 | 41.3 | 74 |
MIS + NPCR | 97.6 | 52.4 | 78.1 |
DMS=Dialysis Malnutrition Score, MIS=Malnutrition Inflammation Score, MUAC=mid-upper arm circumference, NPCR=normalized protein catabolic rate, PEW=protein–energy wasting, SGA=Subjective Global Assessment
Prevalence of PEW based on the Youden index-based cut-offs of the nutritional assessment tools
The prevalence of PEW was calculated for the tools based on the ideal cut-offs determined by the ROC curve and is presented in Supplementary Table 1. The prevalence varied from 35.6% with MUAC to about 78.1% with SGA. The prevalence also varied across males and females between the various tools evaluated in the study. Among males, it ranged from 39.4% (MUAC) to 90.4% (SGA + NPCR), while among females, it ranged between 26.2% (MUAC) to 95.2% (SGA + NPCR) [Supplementary Table 1].
Discussion
The prevalence of PEW among the patients from the index study (56.8%) was the same as that of the estimate from the most recent meta-analysis, which assessed the prevalence of malnutrition in the Indian population as 56.7%.2 The prevalence of PEW was also comparable to that reported by Harvinder et al.,15 that is, 59%. Our estimate was lower than the prevalence calculated in the Indian population by ISRNM 2018 meta-analysis (61%–80%).1 Ours was a government-run center providing dialysis free of cost. Hence, the study population predominantly belonged to lower and lower middle SES.
In the present study, 89.7% of the patients had an actual DEI lower than the required intake. A recent Indian Council of Medical Research study concluded that the Indian diet had lower calorie and protein content than a standard EAT Lancet diet.16 However, currently, there is no Indian data to compare the distribution of DEI and DPI patterns found in our study. Although the unadjusted analysis revealed an association between increasing age and PEW, the association was lost when adjusted for the calorie intake of the patients. Thus, the association found between PEW and increasing age in the univariate analysis might be due to reduced calorie intake.
In our study, MUST was found to have an insignificant predictive capacity for PEW detection among CKD patients. In line with our findings, past studies had concluded MUST as a low-sensitivity tool for detecting PEW in CKD patients on dialysis.17,18 MUAC, as a stand-alone nutritional marker, had a specificity of 79.4%, but a low sensitivity of 47%. As a single anthropometric measure, there is a significant correlation with PEW as observed in other similar studies.19,20 Nevertheless, the lower sensitivity observed in our study warrants a cautious usage of MUAC in the current settings.
DMS and MIS had shown a better sensitivity (80% and 81.9%, respectively). However, the specificity of MIS was higher, which, in turn, increased the accuracy of MIS (82.2%) over DMS (72.6%). This was comparable to data from previous studies.12,13,15 As a stand-alone tool, SGA was found to have lower diagnostic validity than the combination of MIS and DMS. Overall, our findings showed that MIS was better than any other stand-alone or combination of tools in detecting PEW among CKD patients.
NPCR is the most sensitive tool for detecting PEW (90.4%), albeit with a slightly lower accuracy (79.5%) than MIS. Similar findings were obtained in a recent study which compared NPCR with albumin, prealbumin, and transferrin and found NPCR to be a superior marker of nutritional status.21 A combination of NPCR with multiple questionnaires was attempted, and a combination of NPCR with MIS had the best accuracy (78.1%) among the combinations. Yet, stand-alone MIS (82.2%) and NPCR (79.5%) had better accuracy than the combined MIS and NPCR. No data is available in the existing literature to compare the diagnostic validity of the combination of tools.
The current KDOQI 2020 states that serum albumin can be used as an isolated marker for mortality in PEW. Hence, it was tested separately in the present study.4 Serum albumin of the patients had a sensitivity and specificity of 71.1% and 61.1%, respectively, with an accuracy of 69.2%, indicating the scope to be used as a potential marker for PEW. While a recent study compared the simplified creatinine index with PEW identified by ISRNM criteria and found it to be an effective assessment tool,22 the index study found it to be a nonsignificant tool to predict PEW among the studied patients.
Thus, from our study, it can be concluded that NPCR is the most sensitive tool and MIS is the most specific tool to detect PEW. The simplest method was to apply NPCR initially as a screening tool followed by the calculation of MIS, which could predict PEW with higher accuracy. The strength of this study was that it compared multiple nutritional assessment tools in the same cohort with standard conditions. The present study was not devoid of limitations. A higher proportion of males in the study population might have impacted the overall prevalence of the PEW in the current study. The generalizability of the findings is limited owing to the single-centric nature of the study.
Conclusion
PEW was prevalent among 56.8% of CKD patients on hemodialysis, which included predominantly people from a low SES. Lower SES, catheter days, hospitalization days per month and per year, and PHT had a significant association with PEW. Nutritional assessment tools such as MUAC, DMS, MIS, NPCR, and serum albumin were significant predictors of PEW. Among the nutritional assessment tools, MIS had the highest accuracy in predicting PEW.
Acknowledgments
We would like to acknowledge and thank the staff nurses, dialysis technicians, and dialysis students of the Department of Nephrology, Tirunelveli Medical College and Hospital. We thank Dr. Ramprasath, Dr. Karthikeyan, Dr. Nayana, Dr. Harikrishnan, and Dr. Radha Vijayaraghavan for their vital support.
Conflicts of interest
There are no conflicts of interest.
References
- Global prevalence of protein-energy wasting in kidney disease: A meta-analysis of contemporary observational studies from the International Society of Renal Nutrition and Metabolism. J Ren Nutr. 2018;28:380-92.
- [CrossRef] [PubMed] [Google Scholar]
- Estimates of malnutrition associated with chronic kidney disease patients globally and its contrast with India: An evidence based systematic review and meta-analysis. Clin Epidemiol Glob Heal. 2021;12:100855.
- [CrossRef] [Google Scholar]
- A proposed nomenclature and diagnostic criteria for protein-energy wasting in acute and chronic kidney disease. Kidney Int. 2008;73:391-8.
- [CrossRef] [PubMed] [Google Scholar]
- KDOQI clinical practice guideline for nutrition in CKD: 2020 Update. Am J Kidney Dis. 2020;76:S1-S107.
- [CrossRef] [Google Scholar]
- Socioeconomic status scales-modified Kuppuswamy and Udai Pareekh's scale updated for 2019. J Fam Med Prim Care. 2019;8:1846.
- [CrossRef] [PubMed] [Google Scholar]
- Interdialytic weight gain in patients on hemodialysis is associated with dry mouth and thirst. Kidney Int. 2004;66:1662-8.
- [CrossRef] [PubMed] [Google Scholar]
- Second generation logarithmic estimates of single-pool variable volume Kt/V: An analysis of error. J Am Soc Nephrol. 1993;4:1205-13.
- [CrossRef] [PubMed] [Google Scholar]
- Simple formula for calculating normalized protein catabolic rate (NPCR) in hemodialysis (HD) patients. J Am Soc Nephrol. 1993;4:363.
- [Google Scholar]
- Creatinine kinetic modelling: A simple and reliable tool for the assessment of protein nutritional status in haemodialysis patients. Nephrol Dial Transplant. 1995;10:1405-10.
- [Google Scholar]
- The “MUST” Report: Nutritional Screening of Adults? A Multidisciplinary Responsibility?: Development and Use of the “Malnutrition Universal Screening Tool” ('MUST') for Adults Redditch: BAPEN; 2003.
- [Google Scholar]
- Reliability of the 7-point subjective global assessment scale in assessing nutritional status of dialysis patients. Adv Perit Dial. 1999;15:222-5.
- [Google Scholar]
- Prediction of malnutrition using modified subjective global assessment-dialysis malnutrition score in patients on hemodialysis. Indian J Pharm Sci. 2011;73:38.
- [CrossRef] [PubMed] [Google Scholar]
- A Malnutrition-Inflammation Score is correlated with morbidity and mortality in maintenance hemodialysis patients. Am J Kidney Dis. 2001;38:1251-63.
- [CrossRef] [PubMed] [Google Scholar]
- 2022 ESC/ERS Guidelines for the diagnosis and treatment of pulmonary hypertension: Developed by the task force for the diagnosis and treatment of pulmonary hypertension of the European Society of Cardiology (ESC) and the European Respiratory Society (ERS) Eur Heart J. 2022;43:3618-731.
- [CrossRef] [PubMed] [Google Scholar]
- Dialysis malnutrition and malnutrition inflammation scores: Screening tools for prediction of dialysis-related protein-energy wasting in Malaysia. Asia Pac J Clin Nutr. 2016;25:26-33.
- [Google Scholar]
- A comparison of the Indian diet with the EAT-Lancet reference diet. BMC Public Health. 2020;20:812.
- [CrossRef] [PubMed] [Google Scholar]
- The validity of malnutrition universal screening tool (MUST) for nutritional screening in hemodialysis patients. Turkish J Nephrol. 2019;28:109-13.
- [CrossRef] [Google Scholar]
- Sensitive and practical screening instrument for malnutrition in patients with chronic kidney disease. Nutrition. 2020;72:110643.
- [CrossRef] [PubMed] [Google Scholar]
- Assessment of the nutritional status of end-stage renal disease patients on maintenance hemodialysis. Saudi J Kidney Dis Transplant. 2004;15:455-62.
- [Google Scholar]
- Nutritional status of patients on maintenance hemodialysis at Muhimbili National Hospital in Dar es Salaam, Tanzania: A cross-sectional study. J Nutr Metab. 2021;2021:6672185.
- [CrossRef] [PubMed] [Google Scholar]
- Normalized protein catabolic rate is a superior nutritional marker associated with dialysis adequacy in continuous ambulatory peritoneal dialysis patients. Front Med. 2021;7:603725.
- [CrossRef] [PubMed] [Google Scholar]
- Comparison of simplified creatinine index and systemic inflammatory markers for nutritional evaluation of hemodialysis patients. Nutrients. 2021;13:1870.
- [CrossRef] [PubMed] [Google Scholar]